
A Framework for Modelling Dynamic Covid-19 Aerosol
Dispersion and Infection Risk within the Built Environment and
Transportation
FSEG
responded to the COVID19 pandemic by adapting SMARTFIRE to simulate respired
aerosol dispersion; and the EXODUS evacuation model to
simulate physical distancing during pedestrian circulation.
The Problem
- The rapid emergence and global spread of SARS-CoV-2, and evolution of
more transmissible variants, has led to a lack of quantitative
understanding about precise mechanisms of transmission, viral loads and
infectious doses.
- Assessment of Infection Risk and evaluation of mitigations such as air
conditioning & filtration, physical distancing, mask wearing, etc.,
requires models capable of tracking the release and dispersal of
infectious respired droplets and then predicting the risk of infection in
an exposed population.
- Traditional approaches to evaluate Infection Risk often rely on “well
mixed”, or steady-state, assumptions or may use sophisticated modelling
tools in artificially static ways that can be unrepresentative of physical
reality.
- Real world scenarios are influenced by many factors, including:
perturbations caused by dynamic wake flows from people motion; state of
health and movement of people; and degree of immunity in “susceptible”
population – which changes over time due to vaccinations or prior
infection and the prevalence of more/less infectious variants.
A Covid-19 Modelling Framework
The key features of the novel modelling framework are based on
integrating and merging a set of new modelling capabilities with a number of
existing modelling capabilities that were modified and enhanced to address
the new Covid-19 aerosol dispersion application area. These included:
New Modelling Capabilities:
- Respired droplet release model providing appropriate characterisation
of droplet size distribution and suitable expired breath airflow for an
infected person (e.g., when breathing, talking, coughing, etc.);
- Ability to evaluate absolute risk of infection based on exposure to
aerosol droplets at a target volume of interest using a Wells-Riley
approach;
- Support for moving sources i.e., walking sources (infected or “index”
patients);
- Support for stationary or moving targets (i.e., susceptible people);
- The impact of wake flows generated by occupants walking through the
droplet dispersal tracking field, using the immersed boundary method;
- RNG turbulence model to improve flow modelling accuracy;
- Support for air conditioning modelling capabilities to allow the
re-circulation and re-distribution of droplets with the possibility of
droplet culling due to filtration effects (e.g. ordinary or HEPA based
filters) whilst also allowing the arbitrary configuration of the
proportion of fresh and recycled air.
Modified Existing Capabilities:
- Aerosol droplet tracking using Lagrangian particle tracking;
- Droplet evaporation, that is sensitive to humidity and temperature,
with droplet size reduction to “fate” nuclei/fomites with history of
original number of likely virions released in each droplet to allow
infection risk to be deduced from exposure to multiple droplets at
arbitrary times since exhaled;
- Droplet surface deposition, accumulation and persistence;
- New flow pattern definition over the cross section of an inlet.
Adopted Approach using Localised Wells-Riley with CFD Modelling
FSEG implemented a localised form of the Wells-Riley model in
SMARTFIRE[1] using a “quanta” based release allowing calibration of
the many unknowns of Infection Risk against known infection events.
Basic Wells-Riley Equation for Probability of Infection, where D is dose
of infectious agent.
- The CFD model represents respired aerosol droplets using either a
scalar tracer gas transport model or an adapted water mist/droplet
dispersal model to release/track the droplets (sizes from <1 µm to >100 µm
dia.)
- Modelled droplets are carried-by and react-to local environment air
flows, so temperatures and droplet sizes change due to evaporation.
Droplets, composed of respired fluids, attain a fated size due to solids
concentration. Infection Risk is evaluated from droplet residence times in
static or dynamically moving sampling regions.
- Approach also considers dynamics of human behaviour allowing index
patient(s) and susceptibles to move and cause wake flows. This greatly
enhances the sophistication of possible modelling scenarios and gives
better physical agreement with the real-world situation.
- Confidence is gained simulating dispersal events and experiments in
challenging ventilation scenarios (e.g. trains – Infection Risk from
statistical analysis of actual Covid-19 infections; aircraft – Infection
Risk from experimental particle dispersion tracking), showing similar
Infection Risks, trends and conclusions.
Chinese G-Train Infection Risk Modelling
Chinese (long dist.) G-Trains have several ventilation strategies and
large airflow rates.
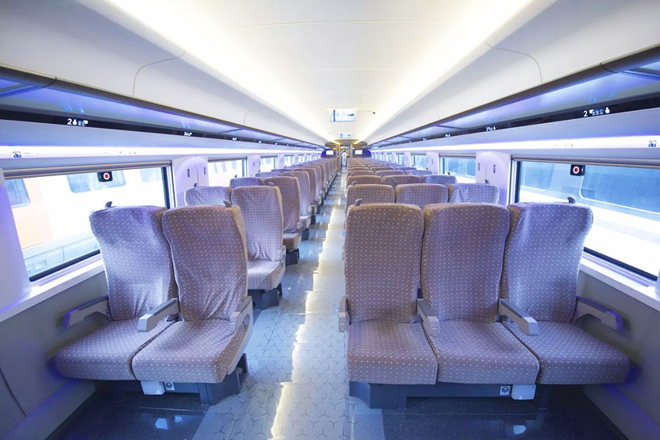
|
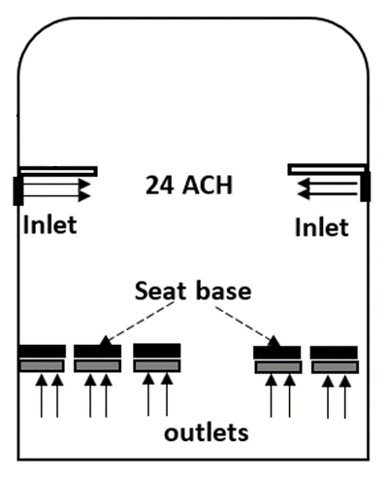
|
Seat Layout in Standard Class G Train Carriage |
Example Ventilation Strategy |
Statistical analysis[2] considered reported infections from many
passenger-journeys during the early stages of the pandemic. This allowed
evaluation of Infection Risk due to proximity to an index patient in various
types of carriage.
CFD Transport Equation for a Scalar
Concentration.
- CFD analysis of Infection Risk at a seat location of interest, using a
scalar dispersal in the carriage ventilation flows, shows good agreement
with trends derived from statistical analysis.
- CFD analysis, being sensitive to local airflows of complex
environments and their interaction with body-heat thermal plumes, provides
an understanding of why certain locations have higher Infection Risk and
how ventilation strategy affects this. Such nuanced analysis is not
possible with simple “well mixed” assumptions that are invalid for complex
train carriage air flows.
- Analysis was extended to evaluate the impact of mask wearing,
demonstrating that, if 90% of passengers wear high efficiency (N95) masks,
overall Infection Risk reduces by 95%.
Inflight Transmission of COVID-19 Based on Aerosol Dispersion Data
FSEG have published an article [3] in Journal of Travel Medicine,
exploring the relationship between exposure time and Infection Risk, based
on Boeing aircraft experimental aerosol dispersion data.
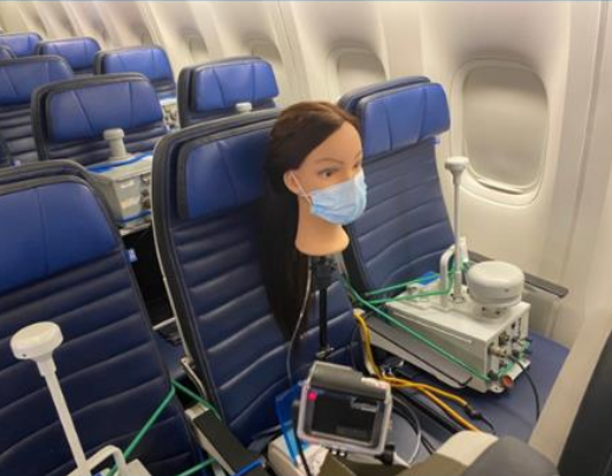
|
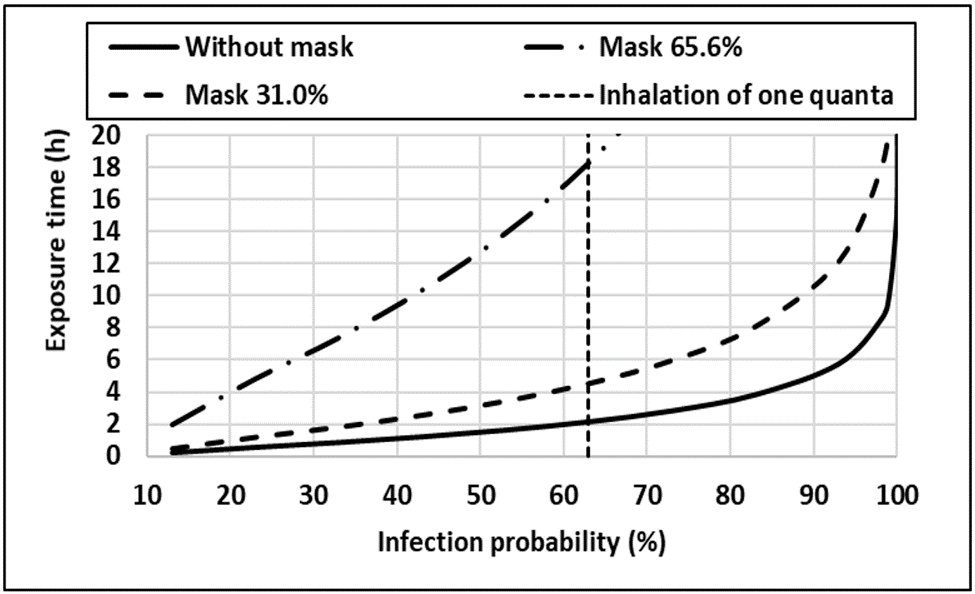
|
Aircraft Aerosol Dispersion Experimental Trial |
Aircraft Infection Probability with Usage of Face Masks |
Summary of FSEG’s conclusions from this Research:
- Time to infection is unrepresentative (and unhelpful) in considering
Infection Risk.
- Long flights (12 hours) can have maximum Infection Risk of 99.6% and
average Infection Risk of 10.8%.
- Efficient masks can reduce Infection Risk by 86%.
Modelling the Dynamics and Impact of People Movement
Although static/steady-state models can be helpful, the real world is
often more complex with transient perturbations. FSEG is developing dynamic
modelling capabilities to allow these complexities to be included in
Infection Risk analysis.
People following through first walker’s respired aerosol cloud (with wake
flows).
Airborne droplets from infected person (mobile source) in air-conditioned
supermarket.
FSEG have also modelled the dynamics of an infected person walking
through, and releasing aerosol droplets in, a ventilated supermarket. This
highlights the impact of low ACH ventilation rates that allow the respired
aerosol droplet cloud to persist for a considerable time and, potentially,
infect others. Droplets can evaporate, deposit on surfaces or deactivate
(over time).
Key Observations
- Infection risk cannot be explained only by large droplets and
fomites. This has been observed at quarantine hotels where shared air
conditioning has resulted in remote infections.
- Mask wearing gives significant benefits in reduction of Infection
Risk.
- High ACH rates give better aerosol clearance, but can cause local
recirculation or air flow paths that actually promote increases in
Infection Risk.
- Dispersion and perturbations, due to people movement, can be
significant.
Challenges During the Project
- The air conditioning recirculation capability presented challenges for
the modelling that have required novel solutions to linked boundary
conditions to represent the way that air conditioning may remove air at
one or more physical locations in a room and re-introduce the recirculated
air at another location – which is especially applicable to passenger
trains and aircraft, where passengers may be exposed to the enclosed
environment for a considerable period of time and to partially
recirculated air containing infectious droplets and nuclei. Droplet
culling and distribution over an outlet (source) had to be resolved for a
variety of use cases where the “behind the scenes” air conditioning
airflows are complex and cannot be modelled.
- One issue that arose was related to the handling of very fast
evaporation of small droplets from respiration. The numerical solver
became extremely slow, to the point of becoming practically unusable, as
it attempted to accurately model this phenomenon. Previously (when solving
purely water mist problems) droplets below a certain % of their original
size were simply removed from consideration by the simulation as their
tiny volumes of water would provide little contribution to suppressing a
fire. Such an approach is not possible with covid particles as the number
of infectious virions in a droplet needs to persist even as the droplet
evaporates to a solid tiny nuclei. Once the problem was identified, for
the smaller droplets, it was assumed appropriate to model them such that
they would instantaneously reach equilibrium diameter. This was found to
be a good approximation as evaporation time was typically less than 1s and
was of the order of 10s.
- A further challenge has been the lack of available data for validation
studies concerning indoor spread of COVID-19. The team have adapted to the
available resources covering droplet dispersal of aircraft and trains, as
well as investigations of superspreading events. Even with these
resources, there are, and have been, significant modelling challenges due
to a lack of information about ventilation characteristics and unknowns
about the respired aerosol generations rates, droplet infectivity and
susceptibility of people and changes due to mutations/variants and
evolving state of population vaccination/natural immunity that make it
difficult to determine representative quanta generation rates. Approaches
have been adopted to address these challenges and the flexibility of the
modelling approach shows the validity of the core modelling assumptions.
It is also challenging to find validation examples that have moving
droplet sources and targets. This is quite typical of advanced modelling
techniques and has been addressed by using validation scenarios that are
more typical of the generally available modelling capabilities in this
application area.
Other Developments
Most capabilities developed/validated in isolation. FSEG are testing full
integration and investigating significant multi-featured and complex
scenarios.
Link with agent-based simulation software EXODUS, enabling movement of
agents attempting to maintain physical distancing, to move sources and
targets for dynamic infection risk analysis in realistic circulation
scenarios.
EXODUS modelling movement (faster than actual) of agents attempting to
maintain physical distancing.
FSEG are continuing to research and develop additional capabilities to
support the Covid-19 aerosol dispersion modelling capabilities. This
includes the development of User Interface capabilities to allow easier
generation and configuration of scenarios and an unstructured meshing
approach to support some of the complex geometries that can be found in
modern buildings and in the fine details of ventilation handling.
Related Work and Links
Presentation (FSEG COVID19 Mitigation Analysis using CFD and Agent Based
Models) describing FSEG CFD model developments relating to dispersion of
respiratory aerosols and COVID19 and applications to transport systems at
the RAMP conference, ‘New Models of Spatial and Social Behaviour in a
Pandemic’ held at Cambridge University, 26-27 May 2021,
Presentation (FSEG COVID19 Mitigation Analysis – Harnessing CFD Fire
Simulation And Agent Based Models), describing FSEG agent based and CFD
model developments associated with COVID19, presented at the Isaac
Newton Institute For Mathematical Sciences, Cambridge University,
Infectious Dynamics Of Pandemics (IDP): Mathematical And Statistical
Challenges In Understanding The Dynamics Of Infectious Disease, Seminar,
Afternoon Session, 14/07/20. Presentation Starts At 01:31:41 Into Video.
Other outreach and promotion activities have used the project work to
target diverse audiences on platforms such as LinkedIn, e.g.:
https://www.linkedin.com/pulse/uninvited-guest-christmas-table-could-infect-two-loved-ed-galea/
https://www.linkedin.com/pulse/fseg-covid-19-mitigation-analysis-harnessing-cfd-fire-ed-galea/
Referenced Work
[1] Z Wang, F Jia, E R Galea, and J H Choi, (2017), “A forensic
analysis of a fatal fire in an indoor shooting range using coupled fire and
evacuation modelling tools”, Fire Safety Journal,
http://doi.org/10.1016/j.firesaf.2017.03.029
[2] Hu et al., "The risk of COVID-19 transmission in train passengers:
an epidemiological and modelling study," Clinical Infectious Diseases, no.
https://doi.org/10.1093/cid/ciaa1057, 2020
[3] Z Wang, E R Galea, A Grandison, J Ewer, F Jia, “Inflight
Transmission of COVID-19 Based on Experimental Aerosol Dispersion Data”,
Journal of Travel Medicine, 2021; https://doi.org/10.1093/jtm/taab023
Acknowledgement
University of Greenwich Innovation Fund – Proof of Concept Development
Award
